The CHC theory in Human Resources
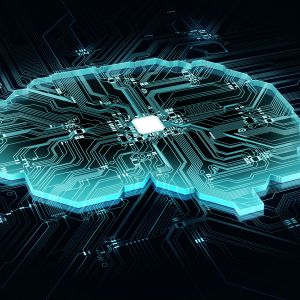
Human skills and abilities are highly diverse. Thus, to be able to heed meaningful differences and disregard marginal dissmilarities between them, an appropriate classification is needed. Obviously, for human characteristics it is unlikely to have a classification like the periodic table, yet there are theoretical approaches based on empirical research, observation and experiments. Probably the broadest of such taxonomies is the Cattel–Horn–Carroll model of cognitive abilities. At PractiWork we consider this as the foundation for new research, innovation and training. In this article, we present the scientific debate about measuring human cognitive abilities in human resource management and demonstrate the benefits of this model. For this we use the study of Schneider and Newman (2015) as a basis.
General vs specific abilities
HR researchers often view cognitive ability as an unidimensional construct since many argue that general cognitive ability is the single most important predictor in personnel selection (Schmidt & Hunter, 1998, 2004) and measuring narrower, specific cognitive abilities is not worth the time (e.g. Hunter, 1986). Applying the unitary approach in personnel selection has practical benefits as it offers a simple and inexpensive way to make not too bad (although quite poor) estimations.
Nevertheless, empirical evidence from factor analysis reveals that unidimensional models of cognitive ability show a notably worse fit than multidimensional or hierarchical models of intelligence. A common argument is that specific cognitive abilities do very little to predict job performance beyond the general g factor. Specific cognitive abilities often have only modest incremental validity in predicting training performance and job performance, however, it is larger than zero and even larger when specific abilities are weighted for each specific job. Moreover, specific abilities account for at least 2% additional variance in job performance and this 2% (or more) increment in job performance prediction can have large monetary benefits.
Schneider and Newman (2015) proposed a compatibility principle of the cognitive ability–job performance relationship which states that general ability predicts general job performance, while specific abilities predict specific job performance. This principle is based on the theoretical work on attitude–behavior compatibility by Ajzen and Fishbein (1977, 1980) and empirical evidence on the predictive validity of specific abilities (e.g. Reeve, 2004; Hunter, 1986; Joseph & Newman, 2010). Besides this, the lack of incremental validity for specific abilities can be caused by criterion measurement issues (e. g. Viswesvaran & Ones, 2002), and according to meta-analytic evidence the criterion validity of specific abilities varies across occupations (e. g. Steel & Kammeyer-Mueller, 2009).
Whereas the first half of compatibility principle (general ability predicts general job performance) has been thoroughly investigated and empirically confirmed, more research is neccessary to assess the second half. To determine exactly which specific abilities are connected to which specific jobs and specific task performance, more theory is needed. It is recommended to classify specific occupations and specific abilities the same way as Hogan and Holland (2003) have classified job criteria based on specific traits they require, Assessing specific abilities in personnel selection and human resources can improve selection decisions and distinctive human capital strategies, in addition to notably improving the ethnic diversity of hiring outcomes (Wee, Newman & Joseph, 2014).
The benefits of measuring specific abilities are emphasized by Agnello, Ryan and Yusko (2015), Becker, Volk and Ward (2015), as well as Coyle and Greiff (2021). The world of work has changed which means a shift in the economy from physical work towards knowledge work in addition to increasing work complexity and cognitive demands. A more complex conceptualization of intelligence and more precise, theoretically-driven assesments are likely to enhance the prediction of performance as they take specific cognitive demands of occupations and specific components of job performance into account (Agnello et al., 2015). Furthermore, specific assessments may improve ethnic diversity in the workplace. Using tranditional intelligence tests – depending on the type of test – can result in large differences across ethnic groups which often translates into lower selection rates for minorities (Hough, Oswald & Ployhart, 2001 as cited in Agnello et al., 2015). However, specific assesments can minimize the differences in selection rates between ethnic groups.
A similar pattern can be seen from the perspective of globalization and cross-cultiral diversity: the more globalizing business world requires the adjustment of selection practices and the elaboration of unified, globally applicable measurement methods Ryan & Tippins, 2009 as cited in Agnello et al., 2015). Intelligence tests focused on measuring general intelligence are often culturally embedded and disregard cross-cultural differences. Specific assesment, on the other hand, may help eliminate this problem: for example the linguistic disadvantages of foreign-born applicants can vanish when using nonverbal measurement methods. Cross-cultural selection methods should also consider the cultural differences in cognitive processing besides the differences of language skills (e.g. Ji, Zhang & Nisbett, 2004 as cited in Agnello et al., 2015).
Becker et al. (2015) focused on the benefits of neuroscientific ability measurements. This is in accordance with the approach of Schneider and Newman (2015), since neurobiological research confirms clear structural distinctions between narrower abilities depicted in the CHC theory (e. g. Nisbett et al., 2012 as cited in Becker et al., 2015). The advantages of neuroscientific specific ability assesments are identical to the aforementioned benefits of non-neuroscientific assesments. Becker et al. (2015) additionally emphasized the role of contextual variables (e. g. job stress, emotional regulation) that influence the realized intelligence and performance in organizational settings.
Coyle and Greiff (2021) highlight the importance of incorporating personality traits, vocational interests and job demands into CHC-based specific assesment methods for a more valid measurement. Besides this, they also stress the minimalizing effect of specific assesments on ethnical differences. Moreover, they support the compatibility principle of Schneider and Newman (2015): general ability predicts general performance, yet assessing specific abilities and identifying their patterns helps achieving a better fit between abilities and environment which leads to more positive outcomes.
The CHC model in the workplace
In the following, we present cognitive abilities based on the Cattel–Horn–Carroll model according to their relevance in personnel selection.
Verbal comprehension and knowledge (Gc), i.e. language ability and general knowledge is among the strongest predictors of job performance (Lang, Kresting, Hülsheger & Lang, 2010), even though it is unlikely that the general knowledge questions are directly connected to performance in most jobs. The reason for this is that people who have extensive knowledge in different fields (e. g. Where is Istanbul?) are more likely to know many other, job-relevant things too. As well as this, people who has learned many things in the past are more likely to to learn new, especially job-relevant, things in the future (Schmidt, Hunter & Outerbridge, 1986).
Some types of domain-specific knowledge (Gkn) are important only in certain professions. The underlying neural mechanisms of informational storage and retrieval are likely the same for general and specialized knowledge. However, it is better to distinguish between them as the effect of general cognitive ability (g) on job performance is mediated by job-specific knowledge and not by general. Specialized knowledge also play a role in complex reasoning: deep background knowledge aids critical thinking and helps to yield positive results in problem solving. In addition, Horn and Blankson (2005) claim that experts in a field can hold large amounts of information in immediate awareness if it is related to their area of expertise, it is called experzise widespan working memory.
Reading/Writing (Grw) and Quantitative knowledge (Gq) would have been considered domain-specific knowledge for hundreds of years. Ont he contrary, nowadays they are considered core skills that are minimal requirements for most occupations. Although true illiteracy is rather rare in industrialized countries, functional illiteracy is fairly common and has substantial negative effects on job performance (Gottfredson, 1997).
Short-term memory (Gsm) has found to be a great predictor of multitasking ability, it mediates the relationship between intellingence and multitasking. Therefore, general ability will not be a useful predictor for jobs with multitasking requirements (Colom, Martínez-Molina, Shih, & Santacreu, 2010). Short-term memory has a great importance in problem-solving processes, however, simple memory span tests show only modest correlations with important outcomes. The reason for this is that this type if tests are highly unrealistic. Working memory tests that require short-term storage and manipulation of information have stronger relationship with important outcomes. In certain jobs, short-term memory can be a strong predictor of job performance, e. g. wait staff must memorize menu items, special offers, dialogue with customers and remember customer orders while checking on other tables (Becker, Volk & Ward, 2015).
Long-term strorage and retrieval (Glr) is not referring to the subject of knowledge but rather tot he process of learning. There are two ability groups within, learning efficiency and retrieval fluency (Schneider & McGrew, 2012). Learning efficiency has a strong relationship with many academic outcomes (McGrew & Wendling, 2010). In personnel selection, learning efficiency is measured in trainability tests in which the aquisition, recall and application of job relevant knowledge and skills are measured. Trainability tests have robust correlations with both traditional cognitive ability tests and long-term assesments of work performance (Roth, Buster & Bobko, 2011). Divergent production tests measuring retrieval fluency may accurately predict creativity (Silvia, Beaty & Nusbaum, 2013).
Processing speed (Gs) is relevant in completing simple tasks at a high speed. According to Ackerman’s (1988) theory of skill acquisition, general cognitive ability (g) predicts performance during the skill learning phase, whereas its predictive validity disappears after practice, particularly in the case of simple tasks. However, processing speed may predict individual differences in skilled performance even long after training, as it reflects the degree to which people can automatize their actions. Fluid reasoning (Gf) is often theoretically indistinguishable from general ability (Horn & Blankson, 2005). It is a crucial precursor to mathematic skills, particularly algebraic reasoning (Floyd, Evans & McGrew, 2003).
From abilities associated with specific sensory modalities, visual-spatial processing (Gv) and auditory processing (Ga) are peculiarly important. The most significant narrow ability within visual-spatial processing is visualization which (counter to other narrow Gv abilities) can be considered as a predictor of carreer choices (Lubinski, 2010) and is a robust predictor of air traffic controllers’ training performance (Ackerman & Kanfer, 1993). One of the most important components of auditory processing is phonemic awareness, its weakness is a strong risk factor of dyslexia and it is associated with poor spelling and difficulty in learning new languages (Caravolas, Hulme, & Snowling, 2001; Comeau, Cormier, Grandmaison, & Lacroix, 1999).
Abilities associated with other sensory modalities generally have greater importance for certain occupations only. Athletes, actors, dancers and mimes must possess a high level of kinesthetic processing (Gk), while olfactory (Go) or gustatory processing (Gg) is essential for perfumers, wine tasters and chefs (Gardner, 1983). Speed of perceptual processing/decision speed is particularly useful in the assesment of reaction time for pilots and in the selection of clerical employees.
References
Ackerman, P.L. (1988). Determinants of individual differences during skill acquisition: Cognitive abilities and information processing. Journal of Experimental Psychology: General, 117(3), 288–318.
Ackerman, P., & Kanfer, R. (1993). Integrating laboratory and field study for improving selection: Development of a battery for predicting Air Traffic Controller success. Journal of Applied Psychology, 78, 413–432.
Agnello, P., Ryan, R., & Yusko, K. P. (2015). Implications of modern intelligence research for assessing intelligence in the workplace. Human Resource Management Review, 25(1), 47–55.
Ajzen, I., & Fishbein, M. (1977). Attitude–behavior relations: A theoretical analysis and review of empirical literature. Psychological Bulletin, 84, 888–918.
Ajzen, I., & Fishbein, M. (1980). Understanding attitudes and predicting social behavior. Englewood Cliffs, NJ: Prentice-Hall.
Becker, W. J., Volk, S., & Ward, M. K. (2015). Leveraging neuroscience for smarter approaches to workplace intelligence. Human Resource Management Review, 25(1), 56–67.
Caravolas, M., Hulme, C., & Snowling, M. J. (2001). The foundations of spelling ability: Evidence from a 3-year longitudinal study. Journal of Memory and Language, 45(4), 751–774.
Colom, R., Martínez-Molina, A., Shih, P. C., & Santacreu, J. (2010). Intelligence, working memory, and multitasking performance. Intelligence, 38(6), 543–551.
Comeau, L., Cormier, P., Grandmaison, E., & Lacroix, D. (1999). A longitudinal study of phonological processing skills in children learning to read in a second language. Journal of Educational Psychology, 91(1), 29–43.
Coyle, T. R. & Greiff, S. (2021). The future of intelligence: The role of specific abilities. Intelligence, 88.
Floyd, R. G., Evans, J. J., & McGrew, K. S. (2003). Relations between measures of Cattell–Horn–Carroll (CHC) cognitive abilities and mathematics achievement across the school-age years. Psychology in the Schools, 40, 155–171.
Gardner, H. (1983). Frames of mind: The theory of multiple intelligences. New York: Basic Books.
Gottfredson, L. (1997). Why g matters: The complexity of everyday life. Intelligence, 24, 79–132.
Hogan, J., & Holland, B. (2003). Using theory to evaluate personality and job–performance relations: A socioanalytic perspective. Journal of Applied Psychology, 88, 100–112.
Horn, J.L., & Blankson, N. (2005). Foundations for better understanding of cognitive abilities. In D. Flanagan, & P. Harrison (Eds.), Contemporary intellectual assessment: Theories, tests, and issues (pp. 41–68) (2nd ed.). New York, NY: Guilford Press.
Hough, L., Oswald, F., & Ployhart, R. (2001). Determinants, detection and amelioration of adverse impact in personnel selection procedures: Issues, evidence and lessons learned. International Journal of Selection and Assessment, 9, 152–194
Hunter, J. E. (1986). Cognitive ability, cognitive aptitude, job knowledge, and job performance. Journal of Vocational Behavior, 29(3), 340–362.
Ji, L. J., Zhang, Z., & Nisbett, R. E. (2004). Is it culture or is it language? Examination of language effects in cross-cultural research on categorization. Journal of Personality and Social Psychology, 87(1), 57–65.
Joseph, D. L., & Newman, D. A. (2010). Emotional intelligence: An integrative meta-analysis and cascading model. Journal of Applied Psychology, 95, 54–78.
Lang, J. W., Kersting, M., Hülsheger, U. R., & Lang, J. (2010). General mental ability, narrower cognitive abilities, and job performance: The perspective of the nested-factors model of cognitive abilities. Personnel Psychology, 63(3), 595–640.
Lubinski, D. (2010). Spatial ability and STEM: A sleeping giant for talent identification and development. Personality and Individual Differences, 49, 344–351.
McGrew, K. S., & Wendling, B. J. (2010). Cattell–Horn–Carroll cognitive-achievement relations: What we have learned from the past 20 years of research. Psychology in the Schools, 47(7), 651–675.
Nisbett, R. E., Aronson, J., Blair, C., Dickens, W., Flynn, J., Halpern, D. F., & Turkheimer, E. (2012). Intelligence: New findings and theoretical developments. American Psychologist, 67(2), 130–159.
Reeve, C. L. (2004). Differential ability antecedents of general and specific dimensions of declarative knowledge: More than g. Intelligence, 32(6), 621–652.
Roth, P. L., Buster, M. A., & Bobko, P. (2011). Updating the trainability tests literature on Black–White subgroup differences and reconsidering criterion-related validity. Journal of Applied Psychology, 96(1), 34–45.
Ryan, A. M., & Tippins, N. (2009). Designing and implementing global selection systems. Malden, MA: Wiley-Blackwell.
Schmidt, F. L., & Hunter, J. E. (1998). The validity and utility of selection methods in personnel psychology: Practical and theoretical implications of 85 years of research findings. Psychological Bulletin, 124,2 6 2 –274.
Schmidt, F. L., & Hunter, J. E. (2004). General mental ability in the world of work: Occupational attainment and job performance. Journal of Personality and Social Psychology, 86(1), 162–173.
Schmidt, F. L., Hunter, J. E., & Outerbridge, A. N. (1986). Impact of job experience and ability on job knowledge, work sample performance, and supervisory ratings of job performance. Journal of Applied Psychology, 71, 432–439.
Schneider, W. J., & McGrew, K. S. (2012). The Cattell–Horn–Carroll model of intelligence. In D. Flanagan, & P. Harrison (Eds.), Contemporary intellectual assessment: Theories, tests, and issues (pp. 99–144) (3rd ed.). New York: Guilford.
Schneider, W. J., & Newman, D. A. (2015). Intelligence is multidimensional: Theoretical review and implications of specific cognitive abilities. Human Resource Management Review, 25(1), 12–27.
Silvia, P.J., Beaty, R.E., & Nusbaum, E.C. (2013). Verbal fluency and creativity: General and specific contributions of broad retrieval ability (Gr) factors to divergent thinking. Intelligence, 41(5), 328–340.
Steel, P., & Kammeyer-Mueller, J. (2009). Using a meta-analytic perspective to enhance job component validation. Personnel Psychology, 62(3), 533–552.
Viswesvaran, C., & Ones, D. S. (2002). Examining the construct of organizational justice: A meta-analytic evaluation of relations with work attitudes and behaviors. Journal of Business Ethics, 38(3), 193–203.
Wee, S., Newman, D. A., & Joseph, D. L. (2014). More than g: Selection quality and adverse impact implications of considering second-stratum cognitive abilities. Journal of Applied Psychology, 99, 547–563.